Dr. Filipovska directly at monika.filipovska@uconn.edu.
Leveraging emerging transportation technologies, new and unconventional data, and mobility innovations, to improve traffic and network operations in transportation systems.
My research focuses on using transportation science and cross-disciplinary analysis approaches to enable small-scale and system-level decision-making for better transportation network and traffic operations. To this end, my research focuses on analyzing, leveraging, and advancing:
- emerging vehicle and infrastructure technologies, including communication and automation capabilities in intelligent transportation systems;
- unconventional and big data, along with advanced data analytics techniques, including applications of data science, information theory, urban informatics, and artificial intelligence;
- innovative perspectives for mobility services and fleet operations, including their intersections with the previous two areas.
My research group is looking to partner in research with collaborators and sponsors across academia, industry, and the government.
Current Projects
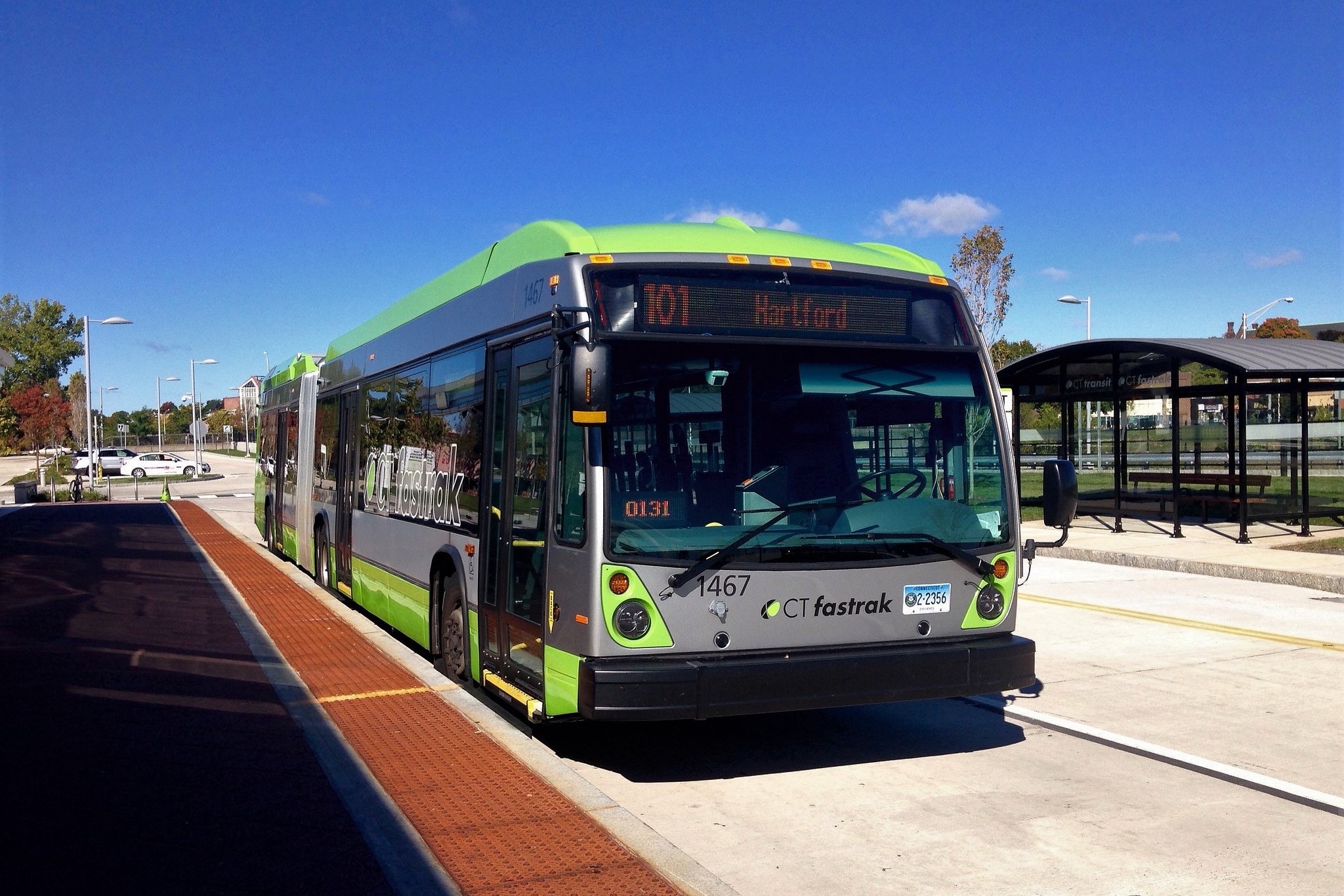
CTfastrak Data Repository for Connected, Automated & Electric Buses
The Connecticut Department of Transportation (CTDOT) is preparing to launch the first American pilot of full-sized, automated buses. In early 2023 CTDOT will deploy three automated and electric buses on CTfastrak, a 9.4-mile limited-access busway linking New Britain and Hartford. In 2020 CTDOT received $2M in Federal Transit Administration (FTA) funds through the Integrated Mobility Innovation program for the project, matched with $500,000 in state funding. New Flyer, Robotic Research, the University of Connecticut, and the Center for Transportation and the Environment are partners to CTDOT on the project.
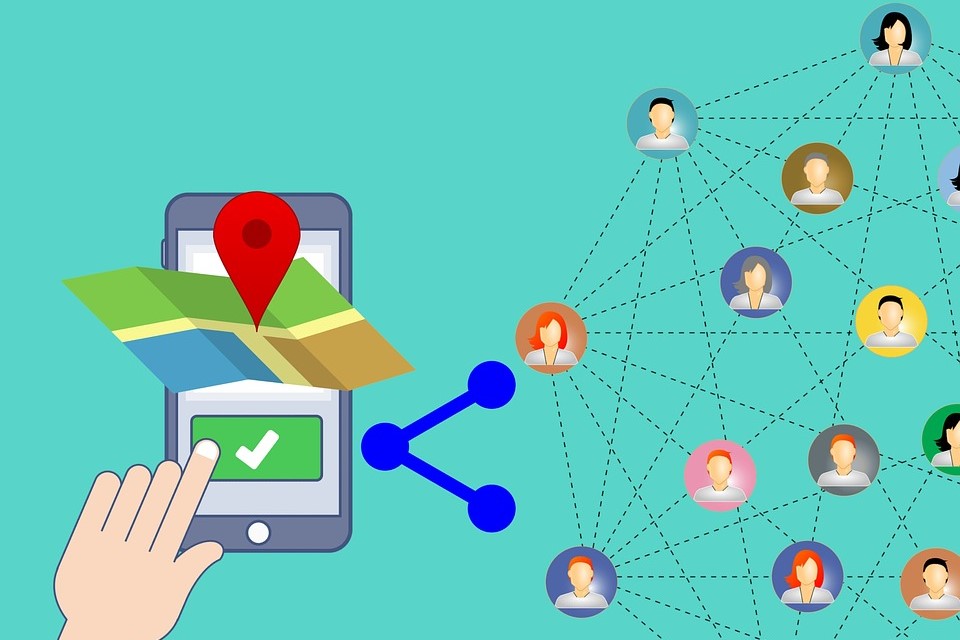
Interpretable Mobility-on-Demand Prediction & Fleet Coordination
The emergence of mobility-on-demand (MOD) services has been redefining personal mobility over the last decade. With new forms of mobility at the advent of Mobility-as-a-Service (MaaS) (e.g., carpool and ridesharing, carsharing, bicycle-sharing systems, etc.), MOD has the potential to revolutionize transportation by promoting responsive and accessible multimodal transportation, and ultimately improving transportation efficiency. In the MaaS space, ride-sharing services, such as Uber, Lyft, Didi, etc. have created a new set of opportunities and challenges. This study focuses on (1) designing interpretable data-driven prediction models to forecast demand for ride-sharing services; and (2) devising efficient operational strategies for the coordination of a city-scale ridesharing vehicle fleet to best satisfy that demand.
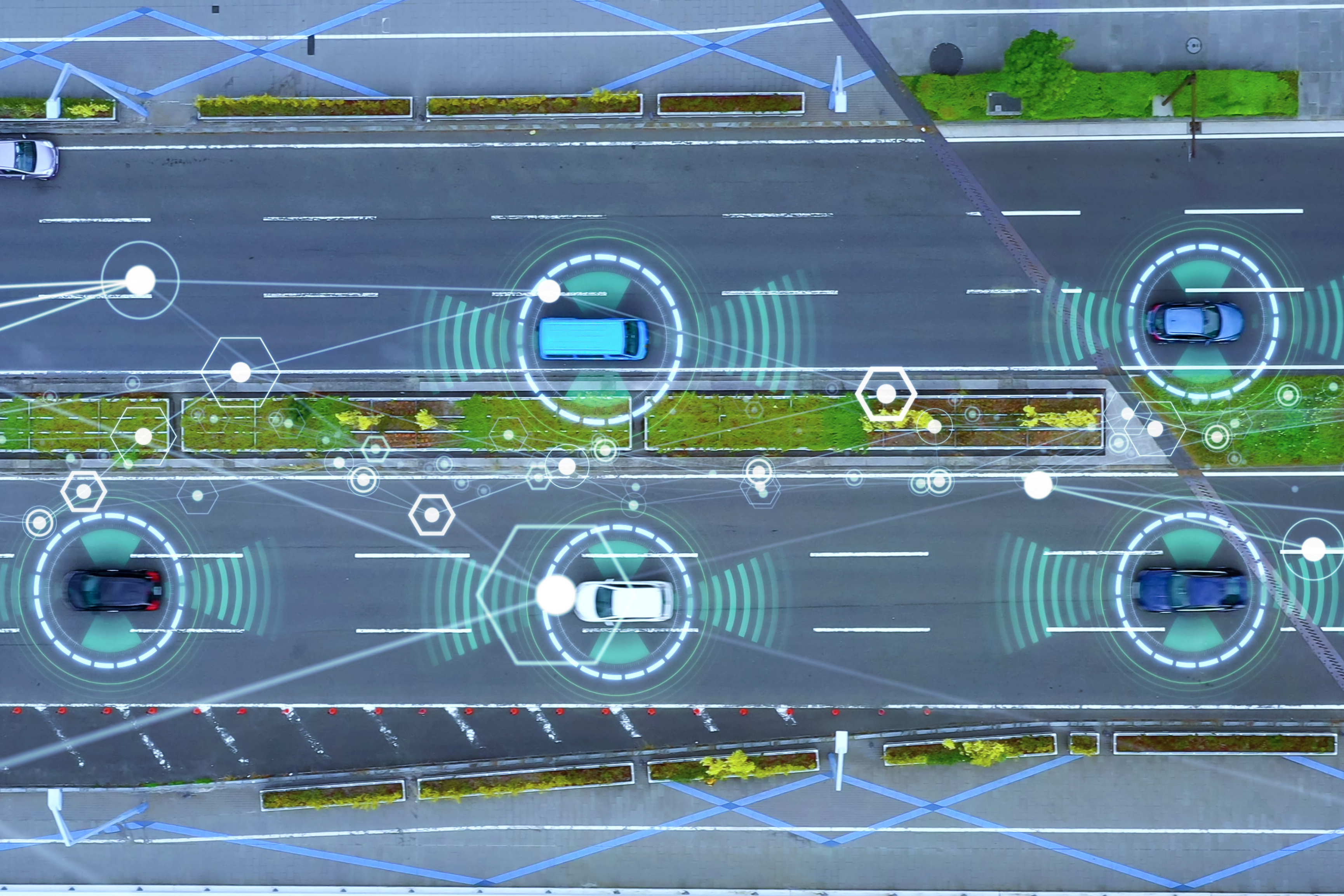
Vehicle Dynamics Analysis Using Connected Vehicle Data
The connected vehicle (CV) data uniquely allows insights into both driving behavior and traffic operations. Using observations across various time periods in Hartford County, this project will focus on data-driven studies to analyze the driving patterns and resulting traffic dynamics under different traffic conditions and control strategies. The analysis will further test the applicability of data fusion approaches to combine CV data with aggregate traffic data to accurately capture, model, and predict traffic conditions. A spatial analysis will be conducted to relate observations of vehicle dynamics to observed crash occurrence towards understanding crash occurrence and the potential of identifying crash prone conditions in real time.